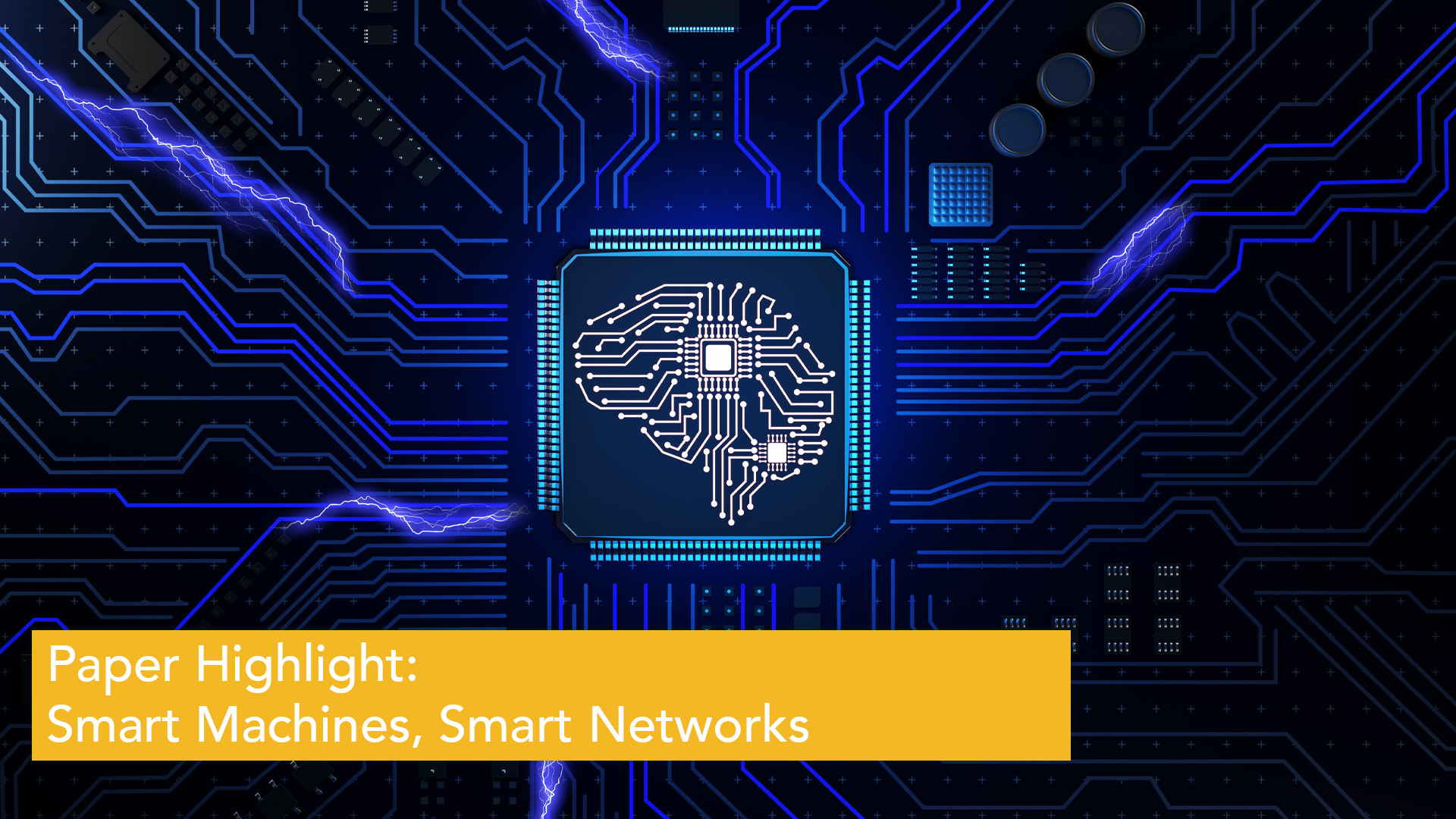
Title: Applications of Distributed Machine Learning for the Internet-of-Things: A Comprehensive Survey
Authors: Mai Le, Thien Huynh-The, Tan Do-Duy, Thai-Hoc Vu, Won-Joo Hwang, and Quoc-Viet Pham
Journal: IEEE Communications Surveys & Tutorials
What’s this paper all about?
Emerging applications and smart services have created numerous opportunities for research and development by enabling the collection of massive amounts of data from end devices (e.g., smartphones and wearables) in various scenarios. In light of this, the application of artificial intelligence and machine learning (ML) has become more apparent, especially in applications in the Internet-of-Things (IoT) like smart healthcare, smart grids, autonomous vehicles, hyperconnected networks, and smart industry. However, conventional ML approaches have raised serious issues since individuals are typically unwilling to share personal data for privacy protection. In addition, the computational limitations of the central server can cause several issues like learning bottlenecks, low efficiency, and single point of vulnerability for data breaches and unauthorised access. Notably, end devices are becoming increasingly powerful, making it feasible to run sophisticated applications (e.g., Mobile Crypto Wallets and Graphic Design Apps) on smart devices, which may have not been possible with a desktop years ago. In this collaborative study, we provide a comprehensive review and insightful discussions on the role of distributed ML for IoT applications and services. We also highlight how our research and lessons can help researchers to outline their future research and to avoid potential pitfalls.
What exactly have you discovered?
We have discovered several insights from the use of various distributed ML techniques and their deployment for different IoT services and applications. First, federated learning, a new paradigm for training ML models across multiple devices without data sharing, is widely recognised for its ability to protect the privacy of private data. When combined with other learning techniques, federated learning can effectively cope with time-varying and large-scale network scenarios. Second, the collection of data from numerous devices and sources necessitates the fusion and curation of data in order to improve learning performance, e.g., localisation accuracy in indoor localisation applications. Third, even with many benefits, distributed ML is not always beneficial, particularly in applications that require real-time learning and security. Fourth, a combination of centralised and distributed learning approaches plays an important role in critical infrastructures. For example, in a complicated manufacturing environment where many collaborative robots are responsible for different tasks, a specialised learning model should be developed to ensure that decisions made by the robots can all satisfy safety constraints while achieving the best mutual performance.
So what?
First, while one of the main benefits of distributed learning in the future Internet is privacy protection, it also faces critical security and privacy issues. It is recommended to further investigate advanced security and privacy techniques, such as methods to secure against quantum computers in the future (i.e., post-quantum cryptography) and approaches to train ML models with encrypted data (i.e., homomorphic computation). Second, distributed learning can be employed on physical devices to enhance user experience and data privacy protection of virtual applications (e.g., Metaverse and holograms). This research area is still in its early stages and thus requires more significant efforts. Third, it is important to develop AI-based resource allocation algorithms that can satisfy multiple metrics like security, privacy, communication cost, complexity, and scalability. Finally, we highlight that it is beneficial to explore the interplay between distributed learning and new AI tools like foundation models and large language models (e.g., ChatGPT and Copilot).
Link to full paper: https://ieeexplore.ieee.org/abstract/document/10597395
CONNECT is the world leading Science Foundation Ireland Research Centre for Future Networks and Communications. CONNECT is funded under the Science Foundation Ireland Research Centres Programme and is co-funded under the European Regional Development Fund. We engage with over 35 companies including large multinationals, SMEs and start-ups. CONNECT brings together world-class expertise from ten Irish academic institutes to create a one-stop-shop for telecommunications research, development and innovation.
ArticlesHomepage FeatureLatest NewsPaper Highlight